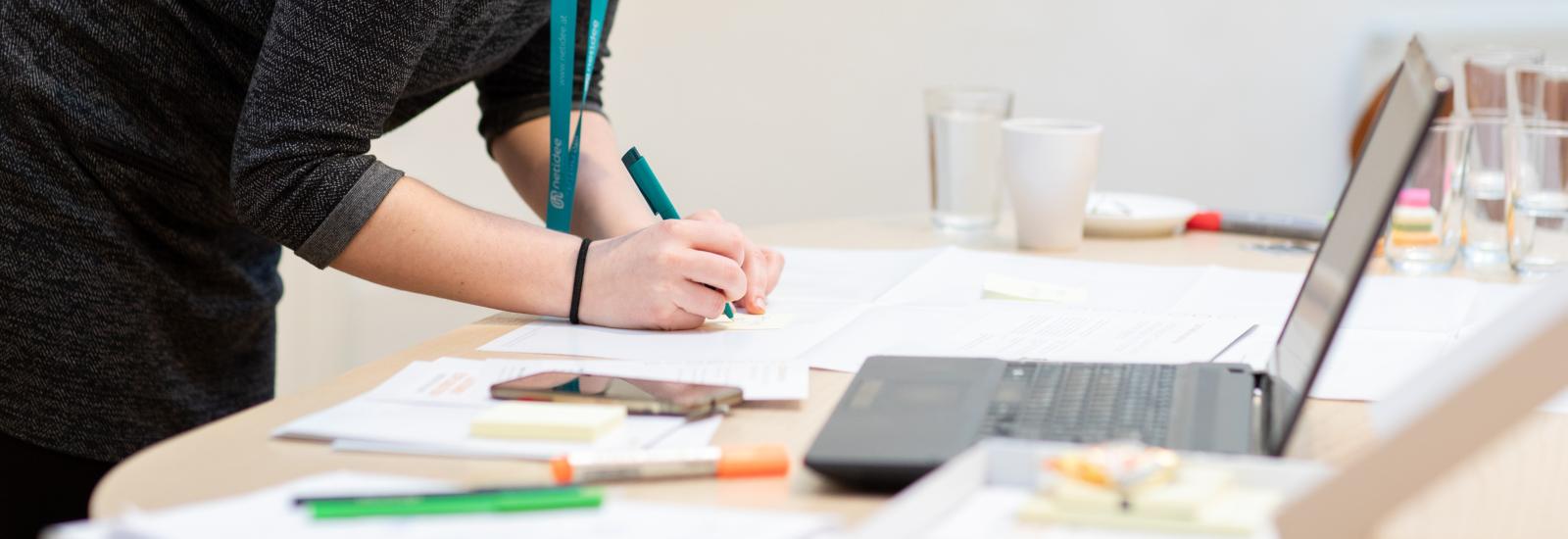
Förderjahr 2018 / Stipendien Call #13 / ProjektID: 3793 / Projekt: Data Management Strategies for Near Real-Time Edge Analytics
To overcome emerging challenges caused by constantly generated data from increasing number of IoT applications, it becomes inevitable to investigate efficient data management strategies performed at the network edge, close to data sources.
In few decades, we will live in data immersive world
Nowadays, sensor-based data are used in a wide set of applications such as eHealth monitoring systems, intelligent traffic control, automated smart homes and buildings, and smart grids. Generally, three steps are required to manage such systems: (1) data are collected through sensors, (2) gathered data are then processed and analyzed, (3) based on the obtained results, certain (near) real-time decisions are taken. Quality of near real-time decisions depends on many factors including data completeness, time response and accuracy of results.
As shown in Figure 1, distant cloud data centers have scalable compute and storage resources, but they are not in the vicinity of IoT end devices that are producing data. As the size of IoT systems is increasing, and consequently producing huge volumes of data, performing data analytics in the cloud may be infeasible due to the strict latency and accuracy requirements coming from IoT gathering layer.
Edge computing - potential solution for emerging IoT applications
To meet emerging requirements from IoT applications in the gathering layer, edge computing becomes a promising solution bringing data processing closer to data sources for decision making processes. Without relying only on the cloud layer, that can be used for resource-intensive batch analytics, use of edge nodes, however, aims to exploit storage and compute resources for near real-time local analytics.
We define edge nodes as locations, between data sources and cloud data centers, in which data can be processed already before reaching the cloud. Accordingly, edge layer include nodes such as edge micro data centers and edge gateways. Edge micro data centers can be considered as ordinary cloud data centers with similar components but designed as smaller systems adjusted for data processing closer to IoT systems and applications.
Upcoming challenges
In order to counter for latency-sensitive IoT applications and to minimize data transmission over the Internet network infrastructure, movement of functionality from the distant cloud to the local edge nodes requires a reliable data management system with support from the IoT gathering layer. We aim to make an Edge Data Management framework able to:
- Facilitate data processing close to the source of data while reducing latency;
- Reduce Internet communication bandwidth usage;
- Deliver accurate decisions for time-sensitive IoT applications.
Nevertheless, there are many challenges in the edge layer. It is necessary to (1) identify all requirements of edge data analytics including limitations and problems for edge data processing, (2) detect and describe necessary components of edge mechanisms, (3) investigate data mining methods for numerosity reduction of sensor data while (4) keeping the high quality and accuracy of edge data processing, for example, in predictive analytics. Proposed algorithms and approaches will be evaluated on relevant IoT data sources. To increase data processing speed, edge computing gives a possibility for challenging distributed analytics among edge nodes. Finally, proposing in-transit data processing principles will lead to efficient interoperability between edge and cloud layer. To validate detected IoT requirements on proposed Edge Data Management framework, it is of great importance to find relevant IoT applications and corresponding data sets for research experiments.