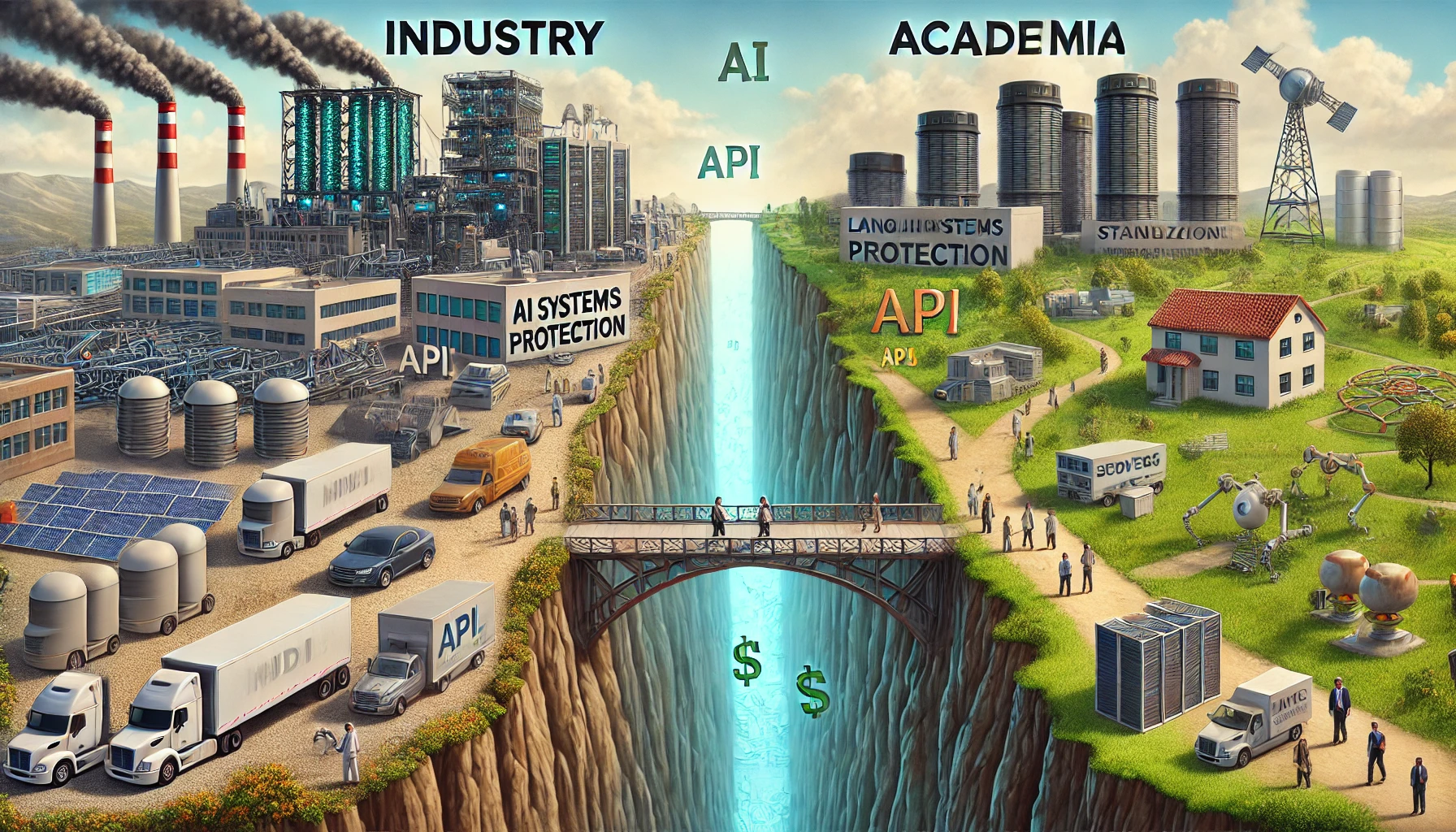
Förderjahr 2023 / Projekt Call #18 / ProjektID: 6872 / Projekt: MONITAUR
The quick rise of AI-based systems unveiled a whole new sector of potential security risks led by Adversarial Machine Learning (AML). These threats include attacks that can compromise the integrity of the systems, like backdoor and poisoning attacks and those that can violate Intellectual Property (IP) rights, like model stealing attacks. While these risks have gathered a lot of attention and potential solutions coming from academic research, recent studies suggest that current industry practices lay far way behind, creating a significant gap between research and reality. In this blog, we highlight some of the issues and concerns of industry practitioners with a particular focus on threats arising from model stealing attacks, which we are countering in MONITAUR.
Real threat?
In several recent studies, several industry practitioners interviewed about AI security stated that losing IP through model stealing attacks raises concerns for them [1] and poses a threat to business [2]. Moreover, some of them admitted that they do not deploy models on end-user devices due to the risk of their models being stolen [1]. Another study that analysed security incidents happening in AI systems reported that a couple of them were model stealing attacks [3]. Although these studies represent only a small fraction of the industry, there is definitely a rising need for IP protection techniques for AI-based systems.
Low priority
Unfortunately, the level of awareness about potential threats caused by AML is relatively low compared to cybersecurity threats [4]. This leads to the industry utilizing mainly non-AML defence approaches without paying enough attention to hazards specific to machine learning applications. Among other reasons for not having AML-specific defences, practitioners state that other cybersecurity threats are more alarming [2] and doubt the capabilities and motivation of an attacker to perform AML-specific attacks [1].
State-of-the-art is not practical
In order to increase the protection level of AI systems, we need reliable, efficient, and reproducible defence approaches. However, taking as an example countermeasures against model stealing studied from both theoretical [5] and practical [6] aspects, we can highlight the following issues:
- Some of the defences are not reproducible or not even implemented
- Defence method or its implementation is inefficient and hence not suitable for real-world applications
- Defence is too invasive (for instance, it requires a trade-off with the model performance)
- Some of the defences are simply broken and can be overcome
Finally, there is no universal or free-lunch protection, so that the risks can not be eliminated completely.
What to do?
Current research calls for the development of best practices to protect AI-based systems within the industry [3]. While establishing best practices requires major effort and global vision, creating open-source protection tools can be a smaller yet impactful step towards a comprehensive solution. This is exactly how we address this problem with MONITAUR, our open-source universal monitoring tool for IP protection of AI-based applications. Keep updated about our progress!
Bibliography:
[1] L. Bieringer, K. Grosse, M. Backes, B. Biggio, and K. Krombholz, “Industrial practitioners’ mental models of adversarial machine learning,” in Eighteenth Symposium on Usable Privacy and Security (SOUPS 2022), Boston, MA: USENIX Association, Aug. 2022, pp. 97–116. [Online]. Available: https://www.usenix.org/conference/soups2022/presentation/bieringer
[2] K. Grosse, L. Bieringer, T. R. Besold, B. Biggio, and K. Krombholz, “Machine Learning Security in Industry: A Quantitative Survey,” IEEE Trans.Inform.Forensic Secur., vol. 18, pp. 1749–1762, 2023, doi: 10.1109/TIFS.2023.3251842.
[3] K. Grosse, L. Bieringer, T. R. Besold, B. Biggio, and A. Alahi, “When Your AI Becomes a Target: AI Security Incidents and Best Practices,” Proceedings of the AAAI Conference on Artificial Intelligence, vol. 38, no. 21, Art. no. 21, Mar. 2024, doi: 10.1609/aaai.v38i21.30347.
[4] F. Boenisch, V. Battis, N. Buchmann, and M. Poikela, "I Never Thought About Securing My Machine Learning Systems: A Study of Security and Privacy Awareness of Machine Learning Practitioners," in Proceedings of Mensch Und Computer 2021, in MuC 21. New York, NY, USA: Association for Computing Machinery, 2021, pp. 520-546. doi: 10.1145/3473856.3473869.
[5] D. Oliynyk, R. Mayer, and A. Rauber, "I Know What You Trained Last Summer: A Survey on Stealing Machine Learning Models and Defences," ACM Comput. Surv., vol. 55, no. 14s, pp. 1 41, Dec. 2023, doi: 10.1145/3595292.
[6] Tushar Nayan, Qiming Guo, Mohammed Al Duniawi, Marcus Botacin, Selcuk Uluagac, and Ruimin Sun, "SoK: All You Need to Know About On-Device ML Model Extraction - The Gap Between Research and Practice," Accessed: Jun. 07, 2024. [Online]. Available: https://www.usenix.org/conference/usenixsecurity24/presentation/nayan