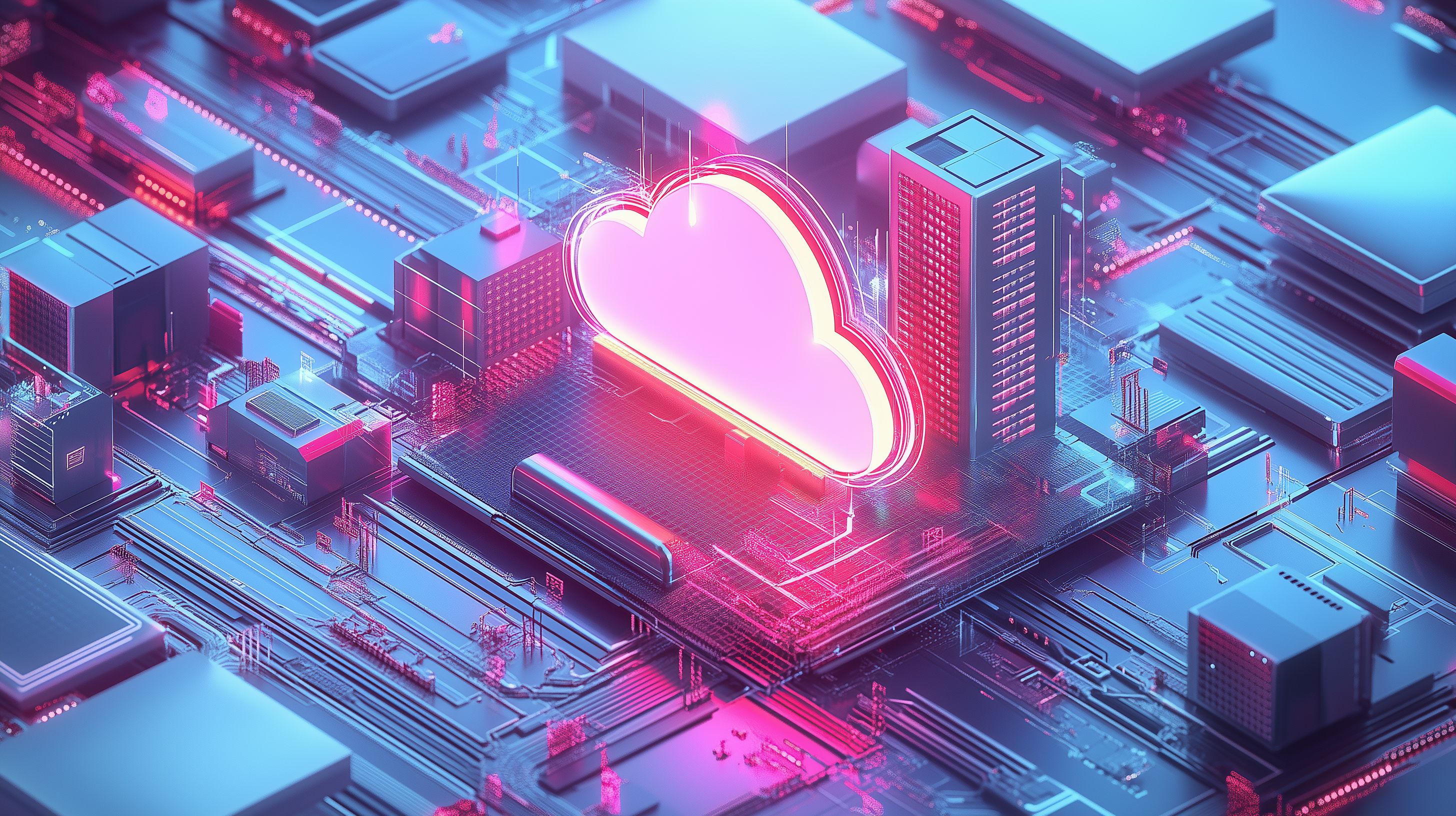
Förderjahr 2024 / Stipendium Call #19 / ProjektID: 7413 / Projekt: Optimizing Hybrid Workflows for Cloud-Based Quantum Computation
A short background about quantum computing
Since its first proposal in the 1980s, quantum computation has developed into an interdisciplinary and active field of research. Today's classical computers, like CPUs, GPUs, and TPUs, operate based on classical physics, which is why they are often referred to as "classical" systems. The fundamental unit of information in these classical devices is the binary digit, or bit in short, which can hold the values 0 or 1. A classical computer encodes bits using two distinct electrical signals, representing the binary values 0 and 1, respectively.
Quantum computers, on the other hand, use quantum systems such as individual photons, ions, or electrons to encode information in quantum bits (qubits). For instance, a qubit can be defined by the horizontal and vertical polarization states of a photon or two distinct energy levels of an ion. Following the physical laws of quantum mechanics, qubits can exist in a superposition of states, allowing them to represent 0, 1, or any combination of these two states "at the same time".
This property of quantum superposition unlocks a completely new computing paradigm, enabling solutions to problems that are intractable for classical computers. However, the transition from the theoretical potential of quantum computing to practical, scalable implementations remains a major challenge that requires research and development of hardware, software, and infrastructure.
The promise of quantum speed-up
One of the most exciting prospects of quantum computing is its potential to outperform classical computers in solving certain "computationally hard" problems—problems that are extremely difficult or time-consuming for classical computers. Algorithms like Shor's algorithm for factoring large integers and Grover's algorithm for unstructured search are prime examples where quantum computers theoretically offer exponential and quadratic speed-ups, respectively, as the problem size increases. Areas of potential applications range from optimization problems to machine learning and the simulation of complex systems. These capabilities could have far-reaching implications for material science, production and logistics, and more.
However, the reality of today's hardware tempers this promise. Current quantum systems operate within the Noisy Intermediate-Scale Quantum (NISQ) era. The noisy and error-prone nature of this generation of quantum computers limits the complexity of computations they can reliably execute. Achieving a quantum advantage, where quantum computers significantly outperform classical computers in practically relevant tasks, such as simulating molecular structures or solving optimization problems for production and logistics, remains an aspirational goal still far in the future.
The NISQ era and cloud-based access
In the NISQ era, quantum hardware is scarce, specialized, and limited in its computational capabilities. While the state of hardware is still in its infancy, building and maintaining a quantum computer requires enormous resources and specialized expertise, which limits access to a select few. To make this technology accessible, companies like IBM, Google, and Amazon Web Services (AWS) provide quantum computing resources via the cloud. This internet-based provisioning of quantum computing resources is often referred to as quantum computing as a service (QCaaS).
Challenges in quantum cloud computing
Through QCaaS, users can access quantum hardware without the need to own the physical infrastructure. Despite the potential of QCaaS, the model also presents challenges. Quantum applications are hybrid in nature, involving classical program execution as well as execution on a quantum device, which is usually a subtask within a larger application workflow. These workflows act as abstraction models, breaking down applications into smaller, interconnected tasks.
Integrating quantum applications with existing classical infrastructure and software systems is one of the primary obstacles. One of the most important issues is the lack of standardized interfaces and programming toolkits for seamless integration. Another crucial issue is the development of effective deployment methodologies for both quantum and classical components within a hybrid quantum-classical computational workflow.
Additionally, the diverse and heterogeneous landscape of quantum computing resources further complicates the practical implementation of QCaaS. The underlying quantum devices vary widely in physical characteristics, such as error rates, supported gate sets, and computational models. Furthermore, cloud providers offer differing configurations, access models, and software ecosystems. This lack of uniformity poses additional challenges to quantum application deployment and performance benchmarking.
Our work addresses some of these challenges and focuses on enhancing the execution of hybrid quantum applications in an as-a-service fashion.