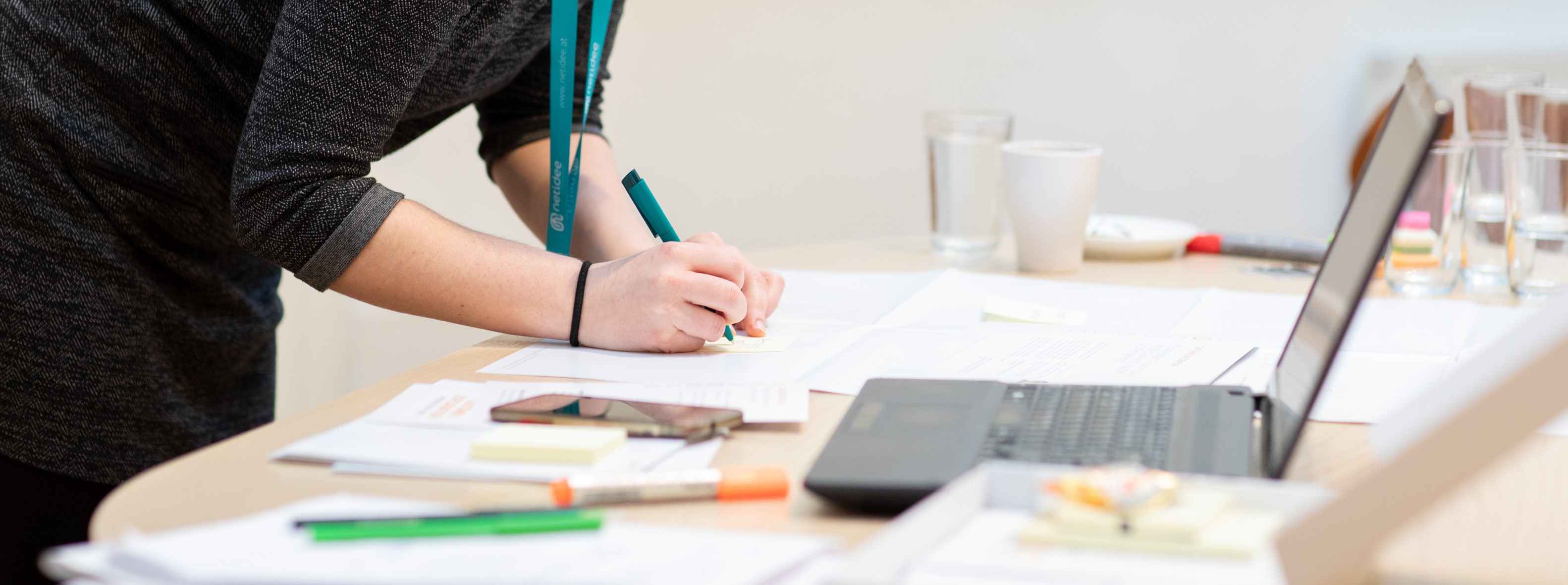
Förderjahr 2018 / Stipendien Call #13 / ProjektID: 3793 / Projekt: Data Management Strategies for Near Real-Time Edge Analytics
With the advent of Internet of Things (IoT), the amount of generated sensor data continues to explode aggravating transfer, storage and processing of big data.
In the IoT concept, Things reference as different types of physical devices, embedded with sensors and actuators that are connected to the Internet. Classical IoT devices generate mostly time series, as a sequence of measurements collected over continuous time. These measurements represent wide set of parameters ranging from electricity consumption, air quality, heart rate or telemetry data gathered at various places during any time interval. With rapid data generation constantly updating big volume of data, current bandwidth capabilities and network capacity cannot easily scale and efficiently handle IoT big data. We are nowadays facing challenges such as:
- critical bandwidth usage;
- inevitable network congestion;
- difficult data integration from distributed data sources;
- expensive transfer of big data to the cloud;
- limited storage capacity of IoT sensors and devices.
Further, raw sensor data are nowadays often incomplete incorporating outliers and missing values due to unexpected situations such as network failures and sensor failures.
Bandwidth fluctuation affects the Quality of Service (QoS) of data-intensive applications
Some of massive consumers of bandwidth that regularly and constantly generate data are: (1) eHealth that monitors the patients’ health status; This includes wearable health devices that send data about vital signs; (2) smart meters and sensors that keep balance between energy demand and supply in smart grids; (3) intelligent traffic monitoring, on-vehicle and roadside sensors. Cars can be powerful source of data that increase bandwidth requirements for collecting and sharing a huge amount of data.
Depending on application QoS requirements (e.g., accuracy, response time) and their criticality, we should explore different approximations in decision making processes, such as:
- Finding acceptable results while applying analytics techniques and making decisions based on raw generated data, that can also be incomplete;
- Exploring what is the representative and relevant data sample that will be selected at runtime making a basis for adaptive analytics and acceptable results;
- Converting or changing raw data to different data representations to meet new features and possibilities for enhancing decision making processes.
Several efforts have been made to cope with huge amount of data at the network edge including methods for reduction of network traffic, data compression techniques and data filtering. However, the impact of different data management strategies on critical data-driven (near) real-time decisions is not taken into account. This is still an open research area and represents an important challenge for future IoT data analytics.
To keep up with a rapid pace of IoT sensors deployment, complex data generation as well as the number of emerging IoT applications and their strict requirements, we need to think about new approaches for decision making processes at the edge of the network.
- Not for every edge deployed system, but definitely yes, as an option in future dynamic IoT environments.