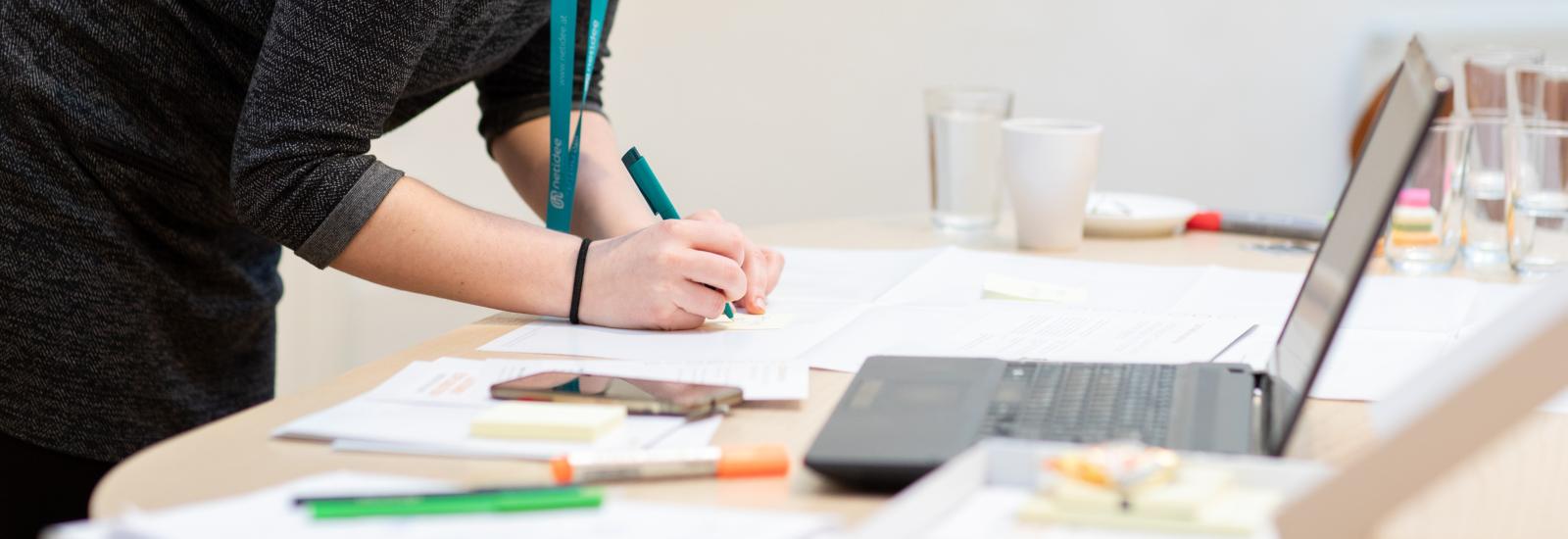
Förderjahr 2018 / Stipendien Call #13 / ProjektID: 3793 / Projekt: Data Management Strategies for Near Real-Time Edge Analytics
Paper "Resilient Edge Data Management Framework" is accepted for publication in the future edition of the scientific journal "IEEE Transactions on Services Computing" (IEEE TSC).
Following the blog series in the January-March quarter of 2019, in this blog post, I give an overview of what the most significant outcomes of the evaluation were.
Data quality impact on the accuracy of the decision-making processes is often neglected in the existing work.
Edge analytics faces us with the problems of making accurate and near real-time decisions that are often based on limited and incomplete data. EDMFrame is designed as a service to enhance data recovery and analytics features. EDMFrame features a generic mechanism for the recovery of multiple gaps in incomplete datasets as well as an adaptive storage management strategy for reducing data stored at the edge while keeping only the data necessary for predictive analytics. These strategies are especially beneficial in scenarios in which applications rely completely on edge computing capabilities rather than consulting cloud services, e.g., in remote edge sites that do not have a connection to the Internet or while being forced to rely on edge resources due to the intermittent connection to the cloud or network congestions.
We evaluate EDMFrame experimentally using six sensor-based time series from smart buildings and homes. Figure 1 shows the main characteristics of datasets.
In the blog post from March 2019, I introduced two methods for data recovery, namely, STR and PRM-based MTR. In the STR scenario, each gap is recovered with a single technique, that uses all data points preceding each gap as input. For the second method, we employ PRMs, used by the recovery mechanism to select the recommended range of required data points and recommended recovery method. Figure 2 shows a PRM for dataset b_1, where for each number of missing values from 1 to 144, the algorithm finds the recommended range of required data points by finding stable clusters and calculating the most accurate cluster. Stable clusters are considered as ones with the highest forecast accuracy (see Definition 6 in the paper). The blue line shows the upper border of the cluster, while the green line its lower. We apply ETS and ARIMA, and the method with the highest accuracy is selected. ETS results are in yellow, while ARIMA in grey. For each selected range of required data points, MAPA values are shown in orange.
We test data recovery with 4 defined gaps using STR as a baseline. In Table 4, we compare running time and MAPE between STR and PRM-based MTR. Results show that EDMFrame is able to recover gaps of various lengths by incorporating recovery cycles, achieving running time of 0,97s on average (with STR), while PRM-based recovery can achieve up to 65,48% less error (e.g., dataset b_1 shown in Figure 2) and only 31,96% more time on average compared to STR. Using PRMs, we can improve at least one objective or both in some cases.
However, current PRM calculation requires a predefined number of consecutive gap lengths, posing obstacles in extreme cases of big gap lengths expected. In future work, we will extend EDMFrame addressing these limitations considering interpolation of ranges and allowing PRM calculation for dynamic IoT cases.
In contrast to existing frameworks, EDMFrame enables (i) a generic data recovery, adaptable to different IoT data sources, and (ii) reliable decision-making for crucial predictive analytics in the context of storage-limited edge nodes.
The full paper is currently available under IEEE Copyright as an Early Access contribution in [1]. A pre-print version is available and listed under the project results (Projektergebnisse) section of the main page of the project.
Publication:
[1] I. Lujic, V. De Maio and I. Brandic, "Resilient Edge Data Management Framework," in IEEE Transactions on Services Computing. doi: 10.1109/TSC.2019.2962016