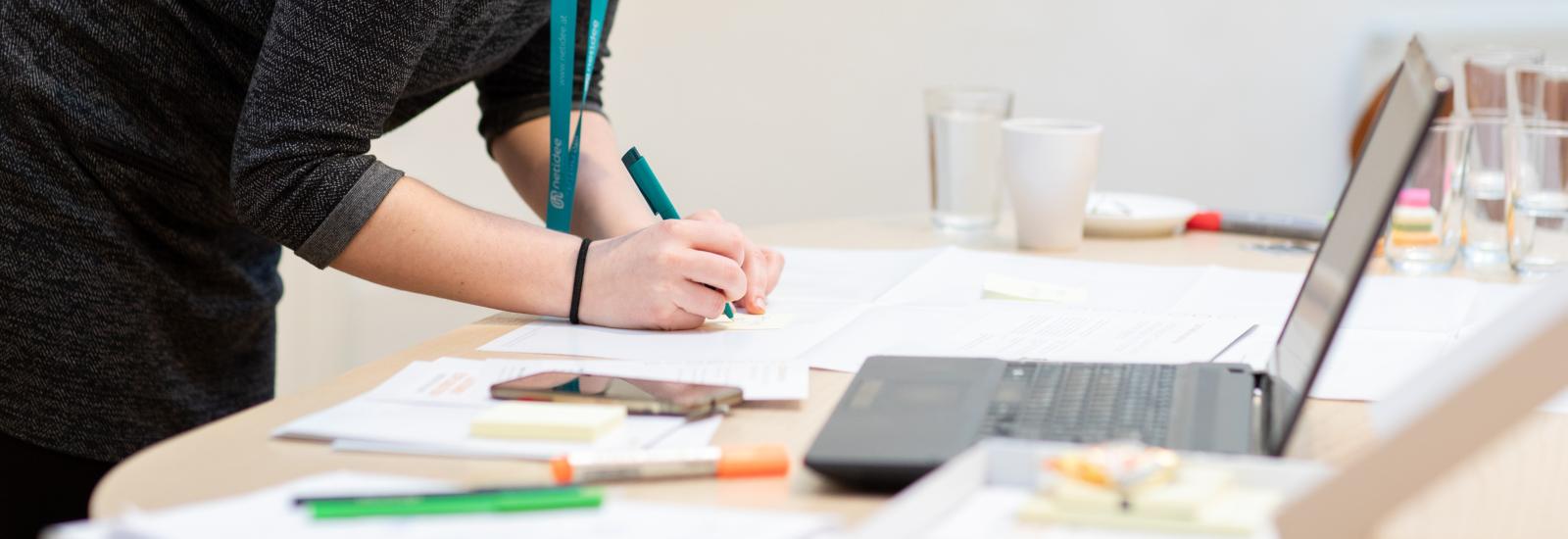
Förderjahr 2023 / Stipendien Call #18 / ProjektID: 6801 / Projekt: Communication and Energy Efficient Edge Artificial Intelligence Framework for Internet of Things
We discuss the limitations of conventional FL systems and lay the groundwork for a hierarchical framework that offers a workaround for navigating these limitations.
The proliferation of IoT devices in the form of smart sensors and edge nodes enables a new set of futuristic applications, such as precision farming and personalized medicine, while simultaneously posing significant challenges to centralized data handling. FL addresses some of these issues by allowing on-device model training and collaborative learning. Although FL has demonstrated its effectiveness in addressing data privacy and communication concerns, it still faces a number of issues, especially related to handling a large number of heterogeneous clients in geographically distributed environments. Moreover, its performance takes a dip whenever encountered with non-IID; the settings in which clients have different data distributions.
The above figure [1] intuitively describes the FL challenges against non-IID and how diverse data distributions at clients lead to different local models, ultimately leading to a global model that is not representative of the clients. In order to deal with the non-IID data challenges various approaches have been proposed. For instance, personalized federated learning (PFL) allows training personalized models for each client. While PFL can be effective for applications with a relatively small number of clients, it faces significant challenges as the number of clients increases due to increased computational and storage costs at the resource-constrained clients.
Hierarchical federated learning (HFL), on the other hand, contrary to conventional FL tries to segregate heterogeneous clients into smaller subsets (clusters) based on some notion of client similarity, trains intermediate edge models for each cluster, and leverages multi-level aggregation to yield, client, cluster, and global models. Since these clusters constitute similar clients and are much smaller in size compared to the total number of clients, enable cluster models to effectively learn the individual cluster characteristics. The training process of HFL can be divided into three main steps; 1) splitting heterogeneous clients into clusters based on similarity, 2) training of local models and aggregation of local models at the edge servers to generate intermediate cluster models, and 3) cloud aggregation using cluster models to yield global model. HFL offers a scalable solution to conventional FL limitations.
References: